In April 2023, an AI quietly emerged that could look at nearly any image and instantly understand what it was seeing. You uploaded a photo. You clicked on a pixel. Instantly, a perfect mask outlined the object beneath, whether it was a hand, a backpack, or the shadow of a tree. It did not matter if the image was a medical scan, cartoon, or street photo.
This was not just image recognition. It was segmentation, and it worked with stunning accuracy and speed. Segment Anything had arrived. With it came a new way of teaching machines how to “see.”
The Problem With Traditional Image Segmentation
Before SAM, segmentation models had to be custom-built for each specific use case. You needed a model for cats, a different one for tumors, and another for road signs. Each model required costly training and tons of labeled data. They often failed when faced with new types of images.
What humans do intuitively—such as pointing and identifying objects—was still difficult for AI. That is where SAM offered a breakthrough.
Its innovation is called promptable segmentation. You give the model a cue, such as a click, a box, or a phrase, and it returns precise object masks.
How Meta Created the Largest Segmentation Dataset Ever
To train SAM, Meta AI built SA-1B, the largest segmentation dataset ever created.
It contains 11 million high-quality images and 1.1 billion object masks. These masks were created through a mix of human feedback and AI self-training.
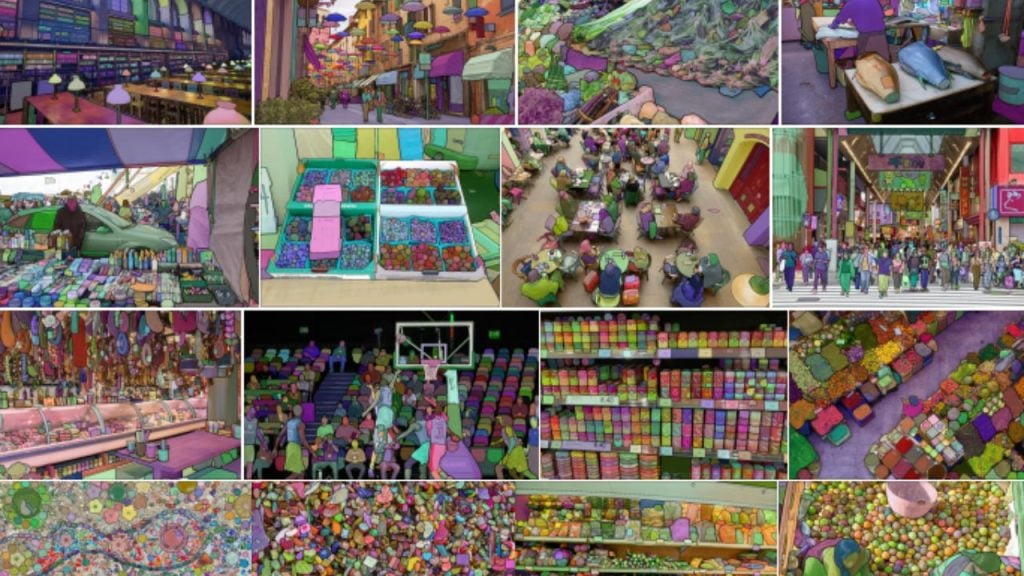
How the Process Worked:
- Assisted Manual Annotation
Humans used early SAM tools to label objects. - Semi-Automatic Annotation
SAM created masks, and humans refined them. - Fully Automatic Generation
The model began generating high-quality masks entirely on its own.
This process formed a feedback loop. The AI improved by generating its own training data, which made it even better over time.
How the SAM Model Works in Real-Time
SAM’s architecture includes three main components:
- A Vision Transformer (ViT-H) to encode the image
- A Prompt Encoder for inputs like points, boxes, or masks
- A Mask Decoder that generates segmentation results in about 50 milliseconds
It does not simply guess. When uncertain, it returns up to three possible masks. This gives users the flexibility to choose the best option.
Since it only needs to process the image once, it is fast enough for real-time interaction—even in a web browser.
Applications of Segment Anything in Design, Robotics, and Medicine
SAM is already transforming several industries.
In medicine, it can segment tumors and tissues in medical scans without retraining. In design, creators can isolate objects or elements with a single click.

In robotics, machines can understand objects they were never trained to recognize. In satellite imaging, SAM can detect roads, rivers, and land features without requiring region-specific models.
Its greatest strength is versatility. It works across many fields with minimal human input.
Is Meta’s Segment Anything Model Fair?
Meta tested SAM across different demographics. They examined performance by skin tone (Fitzpatrick types I–VI), age, and gender presentation. SAM performed consistently across groups.
It even showed slightly better results for older individuals, possibly due to clearer facial features in those images.
However, the team noted that fairness also depends on how SAM is used in downstream applications. High-quality masks are only one part of a fair system.
What This Means for the Future of Computer Vision
SAM does not just classify objects. It segments them. This is a subtle but powerful shift. It moves from knowing what something is to understanding where it begins and ends.
Just as GPT changed how we interact with language, SAM lays the foundation for visual systems that can adapt to any task, without retraining. It is fast, open-source, and ready to use.
Try it now at segment-anything.com.
Final Thought
If we can teach machines to segment anything, can we teach them to perceive the world as we do?
SAM is more than just an AI tool. It represents a new layer of visual intelligence, and its true potential is only beginning to unfold.
Reference: “Segment Anything” by Alexander Kirillov, Eric Mintun, Nikhila Ravi, Hanzi Mao, Chloe Rolland, Laura Gustafson, Tete Xiao, Spencer Whitehead, Alexander C. Berg, Wan-Yen Lo, Piotr Dollár, and Ross Girshick, 2023, arXiv preprint.
DOI: arXiv:2304.02643
TL;DR
Meta’s Segment Anything AI can identify any object in an image using simple prompts. It’s fast, open-source, and already transforming design, medicine, and robotics.